A Data-Driven Approach Towards the Application of Reinforcement Learning Based HVAC Control
Abstract
Refrigeration applications consume a significant share of total electricity demand, with a high indirect impact on global warming through greenhouse gas emissions. Modern technology can help reduce the high power consumption and optimize the cooling control. This paper presents a case study of machine-learning for controlling a commercial refrigeration system. In particular, an approach to reinforcement learning is implemented, trained and validated utilizing a model of a real chiller plant. The reinforcement-learning controller learns to operate the plant based on its interactions with the modeled environment. The validation demonstrates the functionality of the approach, saving around 7% of the energy demand of the reference control. Limitations of the approach were identified in the discretization of the real environment and further model-based simplifications and should be addressed in future research.
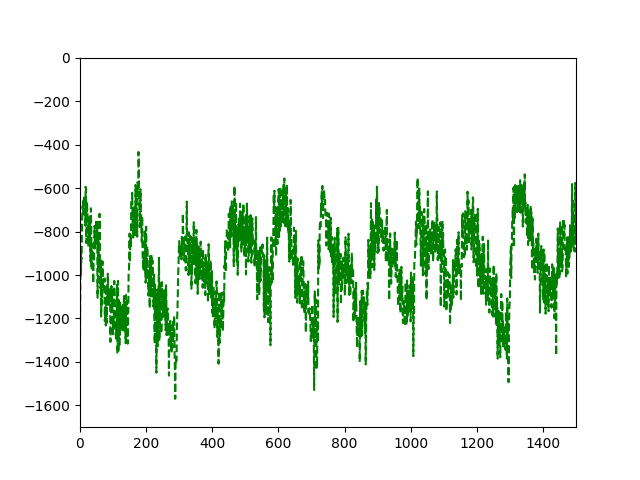
Published
How to Cite
Issue
Section
Copyright (c) 2023 Constantin Falk, Tarek El Ghayed , Ron van de Sand, Jörg Reiff-Stephan

This work is licensed under a Creative Commons Attribution 4.0 International License.