Bayesian Multilevel Models for Count Data
Keywords:
Count Data, Health, Insurance, Dispersion, Multilevel Models.Abstract
The traditional Poisson regression model for fitting count data is considered inadequate to fit over-or under-dispersed count data and new models have been developed to make up for such inadequacies inherent in the model. In this study, Bayesian Multi-level model was proposed using the No-U-Turn Sampler (NUTS) sampler to sample from the posterior distribution. A simulation was carried out for both over-and under-dispersed data from discrete Weibull distribution. Pareto k diagnostics was implemented, and the result showed that under-dispersed and over-dispersed simulated data has all its k value to be less than 0.5, which indicate that all the observations are good. Also all WAIC were the same as LOO-IC except for Poisson in the over-dispersed simulated data. Real-life data set from National Health Insurance Scheme (NHIS) was used for further analysis. Seven multi-level models were f itted and the Geometric model outperformed other model.
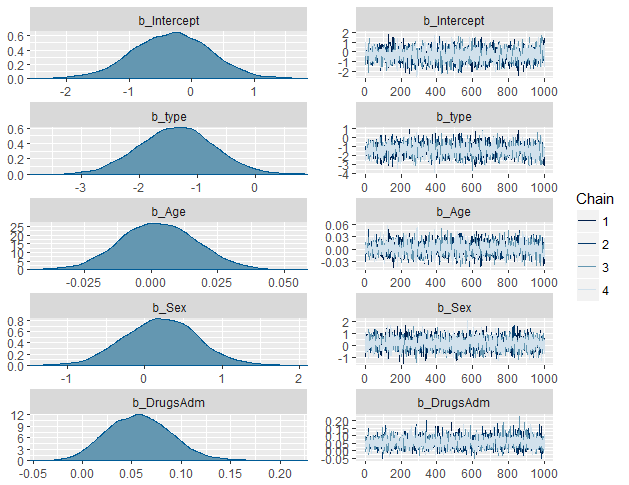
Published
How to Cite
Issue
Section
Copyright (c) 2021 Journal of the Nigerian Society of Physical Sciences

This work is licensed under a Creative Commons Attribution 4.0 International License.