Development of Predictive Model for Radon-222 Estimation in the Atmosphere using Stepwise Regression and Grid Search Based-Random Forest Regression
Keywords:
radon, machine learning, meteorological parameters, atmosphereAbstract
This work develops predictive models for estimating radon (222Rn) activity concentration in the atmosphere using novel grid search based random forest regression (GS-RFR) and stepwise regression (SWR). The developed models employ meteorological parameters which include the temperature, pressure, relative and absolute humidity, wind speed and wind direction as descriptors. Experimental data of radon concentration and meteorological parameters from two observatories of the Korea Polar Research Institute in Antarctica (King Sejong and Jang Bogo) have been employed in this work. The performance of the developed models was assessed using three different performance measuring parameters. On the basis of root mean square error (RMSE), the GS-RFR shows better performance over the SWR. An improvement of 64.09 % and 15.19 % was obtained on the training and test datasets, respectively at King Sejong station. At the Jang Bogo station, an improvement of 75.04 % and 28.04 % was obtained on the training and test datasets, respectively. The precision and robustness of the developed models would be of significant interest in determining the concentration of radon (222Rn) activity concentration in the atmosphere for various physical applications especially in regions where field measuring equipment for radon is not available or measurements have been interrupted.
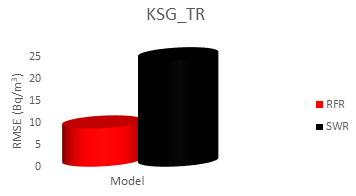
Published
How to Cite
Issue
Section
Copyright (c) 2021 Journal of the Nigerian Society of Physical Sciences

This work is licensed under a Creative Commons Attribution 4.0 International License.