Mamdani-type fuzzy inference system for irrigational water quality
Keywords:
Mamdani fuzzy inference system, USSL diagram, Irrigational water quality groundwater resources, MayiladuthuraiAbstract
This paper aims to evaluate the quality of groundwater resources used for irrigation purposes using a Mamdani-type fuzzy inference system (MFIS). The MFIS is used to resolve ambiguities and uncertainties in economic, social, and natural systems and also facilitates the capture of expert knowledge in ways similar to human reasoning and thought processes. In this study, 20 groundwater samples were collected from various locations within the Mayiladuthurai district, Tamil Nadu, India, between January 2016 and December 2019. These samples underwent physical and chemical analyses to assess the suitability of the collected water resources for irrigation. The analysis utilizes the Mamdani Fuzzy Inference System, which combines values of Electrical Conductivity (EC) and Sodium Adsorption Ratio (SAR). Additionally, methods from the US Salinity Laboratory Staff were also employed. Ultimately, the groundwater quality in Mayiladuthurai is graded for irrigation use by this method. The results indicate that the MFIS reduces imprecision and uncertainty in data handling through the fuzzy membership function. The comparison of irrigation suitability results clearly demonstrates that the proposed MFIS method offers an improved assessment of the irrigation water quality level of the studied groundwater resources.
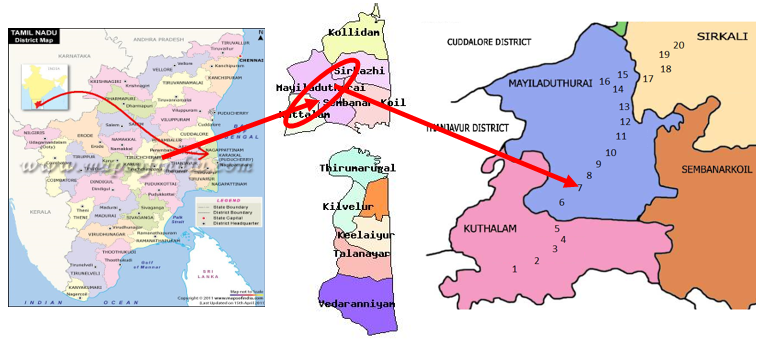
Published
How to Cite
Issue
Section
Copyright (c) 2024 S. Ponsadai Lakshmi, C. Gopi, P. Adwin Jose

This work is licensed under a Creative Commons Attribution 4.0 International License.