Development of a machine learning based fileless malware filter system for cyber-security
Keywords:
Perturbated, Malware, Cyber-attack, FilterAbstract
Over the years, the increased rate of perturbated malware based cyber-attack has presented many challenges and triggered the need for immediate solution all over the world. This was addressed in this paper development of a machine learning based fileless malware filter system for cyber security. Fileless malware which can come inform of Memory Resident Fileless malware or Windows Registry fileless malware are known to have no executable files, resides in the system memory or the windows registry. It doesn’t write any files to disk making it very challenging to detect us[1]ing traditional signature-based methods. It often leverage legitimate tools such as Windows Management Instrumentation and PowerShell to carry out its malicious activities. The methods used were data collection, data extraction, Deep Neural Network (DNN), activation function, training algorithm and classification. The methods were designed using structural and mathematical approaches which employed architectural diagrams, flow charts and self-defining equations to develop the new system. The training of the DNN was done using Gradient Descent Algorithms (GDA) to generate the malware filter algorithm. The filters were implemented with Simulink, tested and validated. The results were also evaluated using Regression (R) and Mean Square Error (MSE analyzer) and it showed R values of 0.9931 and MSE performance also recorded 0.002088Mu. This implied that the filter developed was able to detect and remove malware on the network.
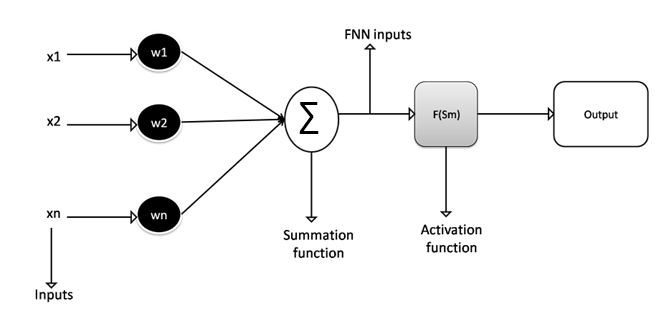
Published
How to Cite
Issue
Section
Copyright (c) 2024 Umaru C. Obini, Chukwu Jeremiah, Sylvester A. Igwe

This work is licensed under a Creative Commons Attribution 4.0 International License.