Optimal control strategies for dynamical model of climate change under real data
Keywords:
Climate change, cost-effectiveness analysis, mathematical model, optimal control, parameters estimationAbstract
Climate change is primarily caused by increasing levels of carbon dioxide (CO$_2$) in the atmosphere, with significant impacts on global temperatures and ecosystems. The interplay between CO$_2$ levels, forest biomass, and temperature highlights how important it is to conserve these vital ecosystems in order to effectively combat climate change. In this study, we construct and analysis the Lotka-Volterra model to explore the interactions between concentration of CO$_2$, photosynthetic biomass density and atmospheric temperature. The results of the model analysis obtained four locally asymptotically stable equilibria under specific conditions and two unstable equilibria. Based on the results of the sensitivity analysis, the most influential parameters affecting changes in concentration number are intrinsic rate of accumulation of CO$_2$ and natural reduction rate of CO$_2$. Next to estimate the model parameters, we employ the least-squares method, enabling us to apply the model to actual temperature data from Surabaya city, Indonesia. The numerical simulation results show that CO$_2$ concentration is expected to range from 400 to 420 ppm, biomass density is estimated to be between 92 and 102 kg/m$^3$, and atmospheric temperature is projected around $28.1^o$C $- 29.1^o$C. Next, using the Incremental Cost-Effectiveness Ratio (ICER) calculation, implementing control strategy in the form of limiting access to private vehicles and reforestation is the best strategy to make the temperature better and cost efficiency.
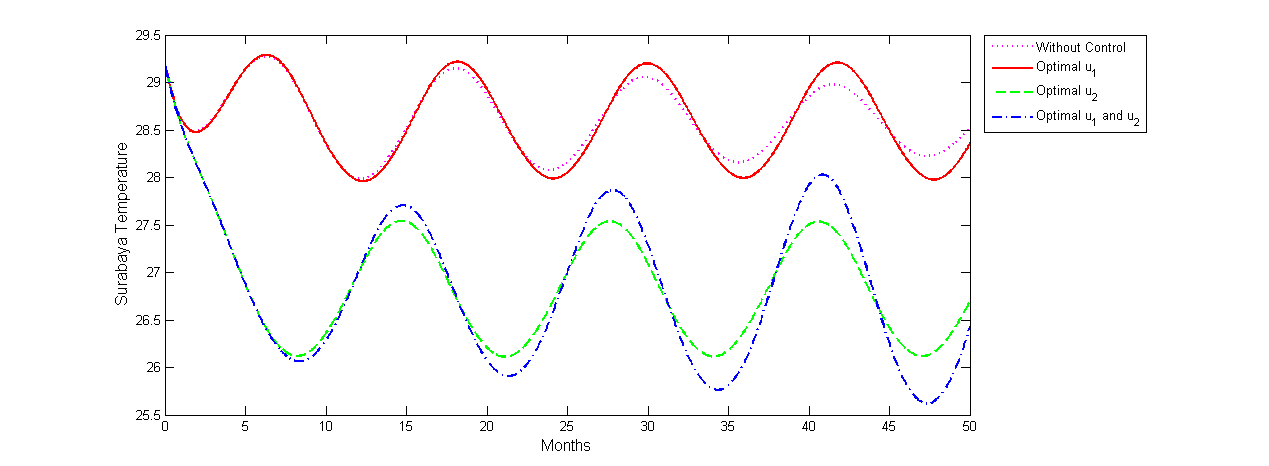
Published
How to Cite
Issue
Section
Copyright (c) 2025 Fatmawati, Faishal F. Herdicho, Nurina Fitriani, Norma Alias, Mazlan Hashim, Olumuyiwa J. Peter

This work is licensed under a Creative Commons Attribution 4.0 International License.