A robust deep learning approach for photovoltaic power forecasting based on feature selection and variational mode decomposition
Keywords:
PV power, Renewable energy, Feature selection, Artificial Neural Networks, ForecastingAbstract
Accurate forecasting of photovoltaic (PV) power is essential for effective grid integration and energy management, particularly in solar-rich regions such as Algeria. This study presents a robust forecasting framework that combines advanced feature selection techniques with deep learning architectures---namely MLP, GRU, LSTM, BiLSTM, and CNN---to enhance daily PV power prediction accuracy. Three feature selection methods---ReliefF, Minimum Correlation, and Minimum Redundancy Maximum Relevance (MRMR)---are employed to identify the most relevant input variables from a dataset collected in the Ghardaia region. Among the selected predictors, Global Solar Radiation (GSR) consistently proves to be the most influential. To further enhance model inputs, Variational Mode Decomposition (VMD) is applied to extract informative Intrinsic Mode Functions (IMFs) from the selected features. A comparative evaluation of the models indicates that recurrent neural networks, particularly GRU and LSTM, deliver superior performance across various metrics, including RMSE, MAE, nRMSE, nMAE, R², and the correlation coefficient. The GRU model achieves the best results, with an RMSE of 3.246 and an R² of 0.9550 using five IMFs. These findings highlight the effectiveness of integrating optimal feature selection, signal decomposition, and deep learning for reliable PV power forecasting. The proposed hybrid approach provides a practical and scalable solution for enhancing energy planning and operational efficiency in high-solar-potential regions.
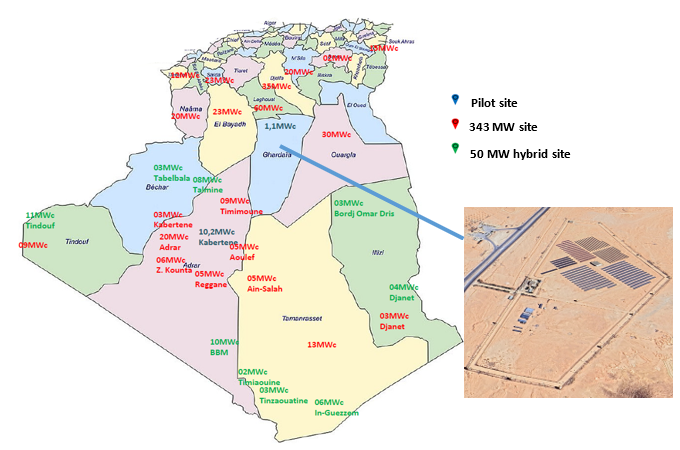
Published
How to Cite
Issue
Section
Copyright (c) 2025 Mokhtar Ali, Abdelkerim Souahlia, Abdelhalim Rabehi, Mawloud Guermoui, Ali Teta, Imad Eddine Tibermacine, Abdelaziz Rabehi, Mohamed Benghanem

This work is licensed under a Creative Commons Attribution 4.0 International License.