On the cluster of the families of hybrid polynomial kernels in kernel density estimation
Keywords:
Kernel density estimation, cluster of hybrid kernels, Classical polynomial kernels, global error, Monte Carlo SimulationAbstract
This study introduces a novel cluster of hybrid polynomial kernel families, designed to achieve significantly lower asymptotic mean integrated squared error compared to traditional kernels. These hybrid kernels are developed by heuristically combining classical polynomial kernels using probability axioms. An in-depth analysis of error propagation within these kernels is conducted, utilizing both simulation experiments and real-life datasets, including the Life Span of Batteries and COVID-19 datasets. The findings consistently demonstrate that the proposed hybrid kernels outperform their classical counterparts in various density estimation tasks across different distribution types and sample sizes. This research highlights the potential of hybrid polynomial kernels to enhance accuracy in density estimation, advocating for their adoption in statistical modelling and analysis.
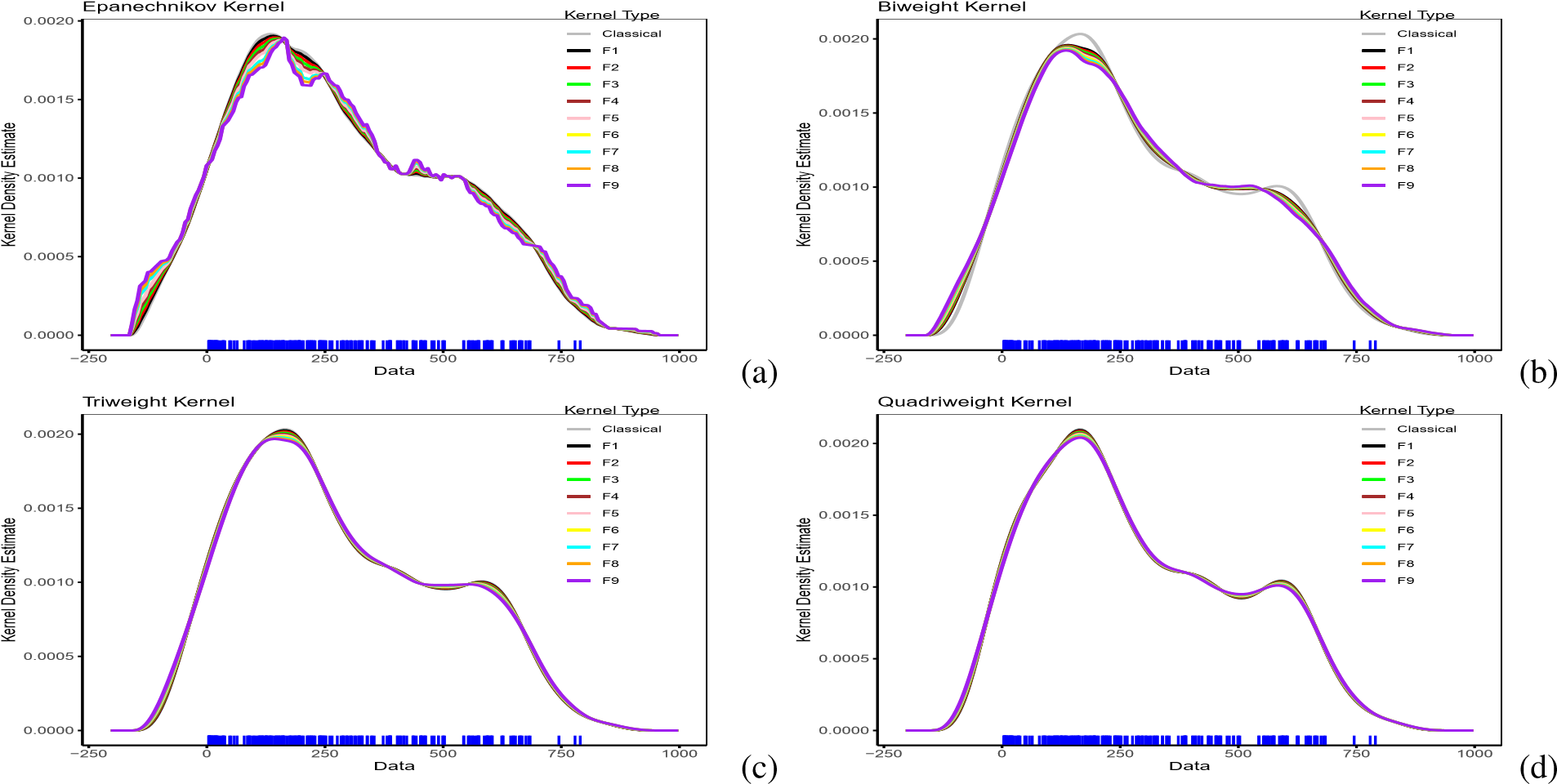
Published
How to Cite
Issue
Section
Copyright (c) 2024 Benson Ade Eniola Afere

This work is licensed under a Creative Commons Attribution 4.0 International License.