A machine learning sentiment classification of factors that shape trust in smart contracts
Keywords:
Blockchain, Cybersecurity,, Machine learning, Smart contracts, Sentiment analysis, TrustAbstract
Smart contracts have attracted significant attention within the blockchain ecosystem due to their ability to automate agreements when specific pre-defined conditions are met. However, concerns about the reliability of smart contracts persist due to potential vulnerabilities and unexpected outcomes. This study seeks to examine the perception of various stakeholders in the blockchain community, including developers, regulators, investors, researchers, auditors, and enthusiasts to understand the factors that influence trust in smart contracts. Data was gathered from 213 respondents through a survey administered across two blockchain communities. The responses were analyzed to identify key factors shaping trust in smart contracts within the blockchain space. The study identified five critical factors that significantly affect trust perceptions: Perceived Security Measures (PSM), Perceived Design Practices and Developer’s Reputation (PDR), User Experience (UX), Perceived Social and Psychological Influence (PSP), and Perceived Regulatory Compliance and Continuous Improvement (PRC). Additionally, machine learning algorithms namely Support Vector Machine, Decision Tree, Logistic Regression, and Naive Bayes were applied on open-ended responses to conduct sentiment analysis, providing deeper insights into the perceptions of trust in blockchain smart contracts. The results revealed that Logistic Regression classifier outperformed the other models in analyzing trust levels in smart contracts.
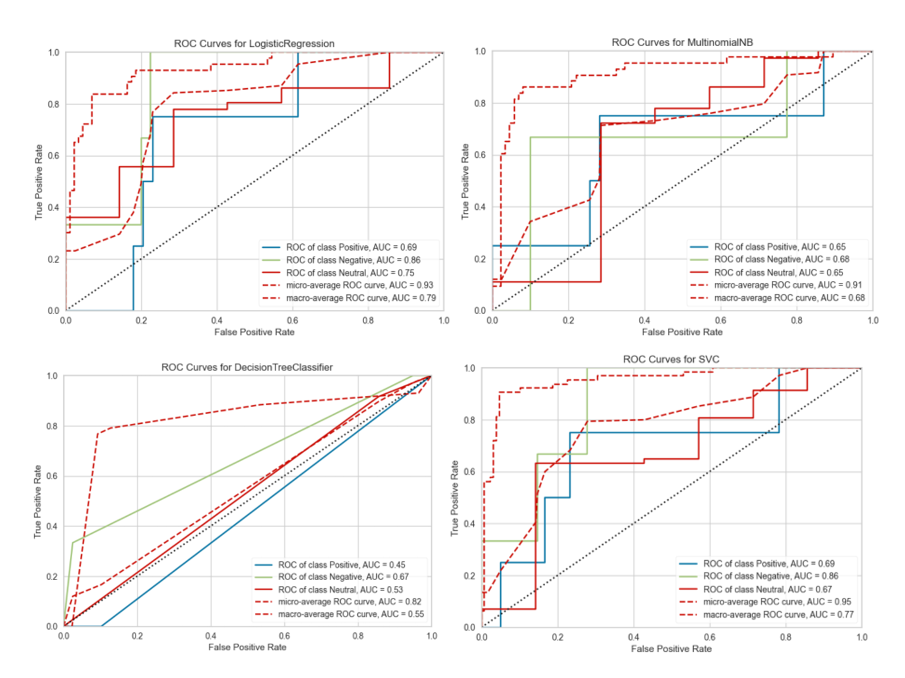
Published
How to Cite
Issue
Section
Copyright (c) 2024 Unyime Ufok Ibekwe, Uche M. Mbanaso, Nwojo Agwu Nnanna, Umar Adam Ibrahim

This work is licensed under a Creative Commons Attribution 4.0 International License.