Optimizing precision farming: enhancing machine learning efficiency with robust regression techniques in high-dimensional data
Keywords:
lasso, Ridge, M-estimation, MM-estimation, Robust RegressionAbstract
Smart precision farming leverages IoT, cloud computing, and big data to optimize agricultural productivity, lower costs, and promote sustainability through digitalization and intelligent methodologies. However, it faces challenges such as managing complex variables, addressing multicollinearity, handling outliers, ensuring model robustness, and enhancing accuracy, particularly with small to medium-sized datasets. To overcome these obstacles, reducing retraining time and resolving the complexity issue is essential for improving the machine learning algorithm’s performance, scalability, and efficiency, especially when dealing with large or high-dimensional datasets. In a recent study involving 435 drying parameters and 1,914 observations, two machine learning algorithms - Ridge and Lasso - were employed to analyze and compare the impact of two variable selection techniques, specifically the regularization methods Ridge and Lasso, before and after addressing heterogeneity in highly ranked variables (50, 100, 150, 200, 250, 300). Additionally, robust regression methods such as S, M, MM, M-Hampel, M-Huber, M-Tukey, MM-bisquare, MM-Hampel, and MM-Huber were applied. The results demonstrated that the robust methods, when applied to Ridge and Lasso, achieved the highest efficiency, with the smallest values for MAPE, MSE, SSE, and the highest R2 values, both before and after accounting for heterogeneity. As a result of the study, the best models are the Ridge model with the MM bisquares before heterogeneity, the Ridge model with the MM method after heterogeneity, and the Lasso model with the MM method before heterogeneity and the Lasso model with MM Hampel after heterogeneity.
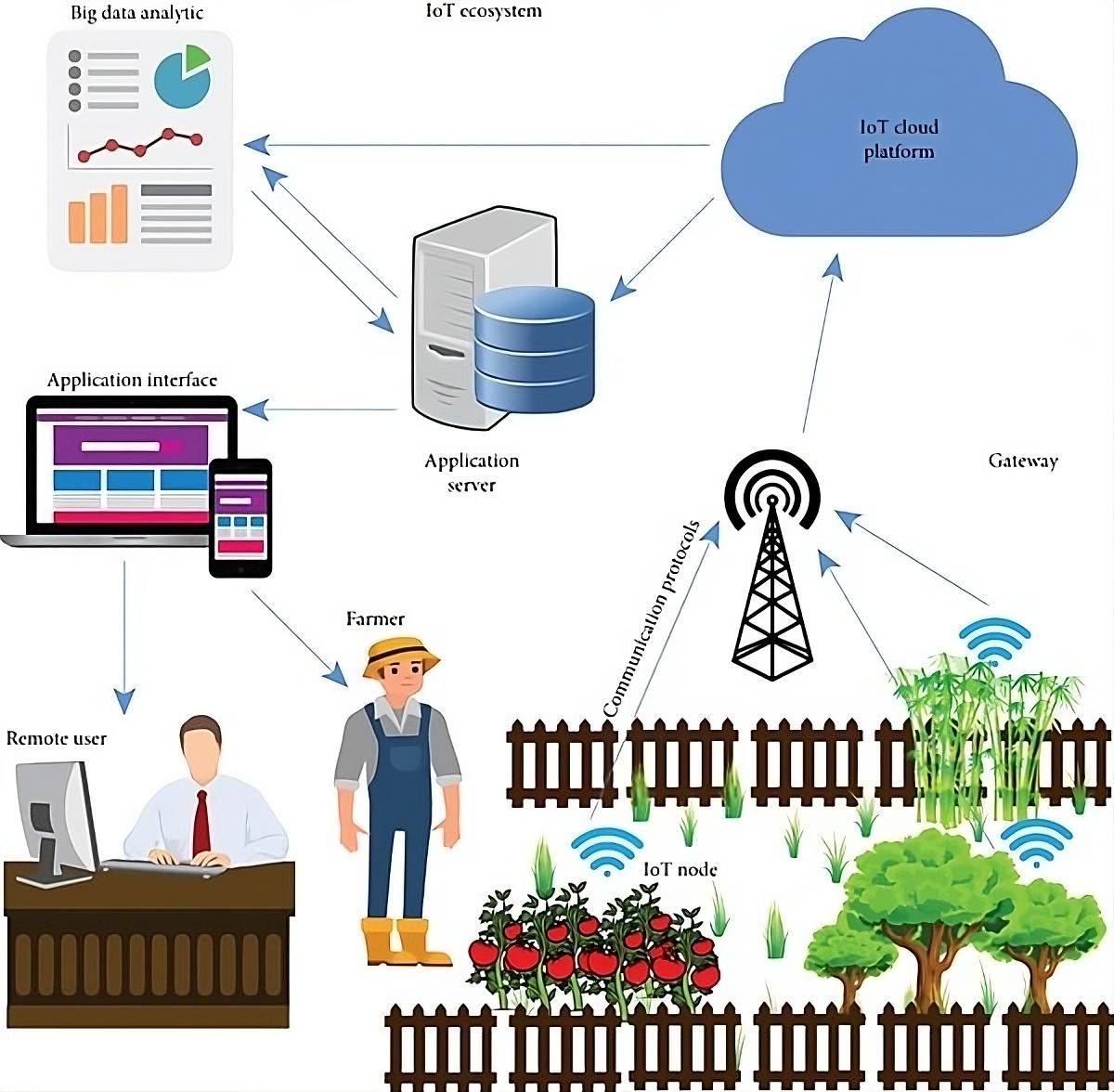
Published
How to Cite
Issue
Section
Copyright (c) 2024 Nour Hamad Abu Afouna, Majid Khan Majahar Ali

This work is licensed under a Creative Commons Attribution 4.0 International License.