The effect of imbalance data mitigation techniques on cardiovascular disease prediction
Keywords:
Imbalance dataset, Cardiovascular disease prediction, SMOTE-TOMEK, Marchine learning, Overfitting and UnderfittingAbstract
The prevalence of class imbalance is a common challenge in medical datasets, which can adversely affect the performance of machine learning models. This paper explores how several data imbalance mitigation techniques affect the performance of cardiovascular disease prediction. This study applied various data balancing techniques on a real-life cardiovascular disease (CVD) dataset of 1000 patient records with 14 features obtained from the University of Abuja Teaching Hospital Nigeria to address this problem. The data balancing techniques used include random under-sampling, Synthetic Minority Over-sampling Technique (SMOTE), Synthetic Minority Oversampling-Edited Nearest Neighbour (SMOTE-ENN), and the combination of SMOTE and Tomek Links undersampling (SMOTE-TOMEK). After applying these techniques, their performance was evaluated on seven machine learning models, including Random Forest, XGBoost, LightGBM, Gradient Boosting, K-Nearest Neighbours, Decision Tree, and Support Vector Machine. The evaluation metrics used are precision, recall, F1-score, accuracy, and receiver operating characteristic-area under the curve (ROC-AUC). Learning curve plots were also used to showcase the impact of the different data balancing techniques on the challenges of overfitting and underfitting. The results showed that the application of data balancing techniques significantly enhances the performance of machine learning models in heart disease prediction and effectively addresses the challenges of overfitting and underfitting with SMOTE-TOMEK, yielding the best-balanced fit as well as the highest precision, recall, F1-score, accuracy of 92%, and ROC-AUC of 96% on the Lightweight Gradient Boosting Machine (LightGBM) model. These results underscore the critical role of data balancing in predictive modelling for heart disease and highlight the effectiveness of specific techniques and models in achieving accurate, more reliable, and generalised predictions.
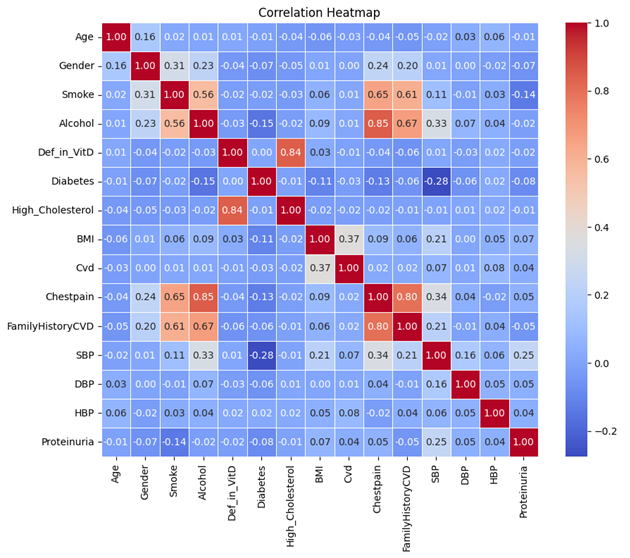
Published
How to Cite
Issue
Section
Copyright (c) 2025 Raphael Ozighor Enihe, Rajesh Prasad, Francisca Nonyelum Ogwueleka, Fatimah Binta Abdullahi

This work is licensed under a Creative Commons Attribution 4.0 International License.