A Dai-Kou-Type method with image de-blurring application
Authors
- K. Ahmed Department of Mathematical Sciences, Bayero University, Kano, Nigeria
- M. Y. Waziri Department of Mathematical Sciences, Bayero University, Kano, Nigeria
- A. S. Halilu Department of Mathematics, Sule Lamido University Kafin Hausa, Nigeria
- I. A. R. Moghrab Information Systems and Technology Department, Kuwait Technical College, Kuwait
- H. Abdullahi Department of Mathematics, Sule Lamido University Kafin Hausa, Nigeria
- S. M. Ibrahim School of Quantitative Science, Universiti Utara Malaysia, Sintok, 06010, Kedah, Malaysia
- Y. B. Musa Department of Mathematics, Sule Lamido University Kafin Hausa, Nigeria
- S. Murtala Department of Mathematics, Federal University, Dutse, Nigeria
- A. M. Awwal Department of Mathematics, Gombe State University, Gombe, Nigeria
- A. Sambas Faculty of Informatics and Computing, Universiti Sultan Zainal Abidin, Campus Besut, 22200 Terengganu, Malaysia
Keywords:
Nonlinear monotone systems, Spectral parameter, Image de-blurring, Convex constraintAbstract
By exploiting nice attributes of three-term conjugate gradient (TTCG) methods and the efficiency of the Dai-Kou scheme, this paper proposes a spectral class of Dai-Kou-type methods for monotone systems with convex constraints. The scheme combines a modified Dai-Kou search direction with the projection method and can best be described as an adaptation of the Dai-Kou method to nonlinear systems. An important contribution of the scheme is its application to image de-blurring. The method is shown to converge globally under mild assumptions. Furthermore, test results of some numerical experiments suggest that the proposed approach outperforms three recent schemes for convex constrained monotone systems.
[1] W. L. Cruz & M. Raydan, “Nonmonotone spectral methods for large-scale nonlinear systems”, Optimization Methods and Software 18 (2003) 583. https://doi.org/10.1080/10556780310001610493.
[2] G. A. Hively, “On a class of nonlinear integral equations arising in transport theory”, SIAM Journal on Mathematical Analysis 9 (1978) 787. https://doi.org/10.1137/0509060.
[3] S. P. Dirkse & M. C. Ferris, “A collection of nonlinear mixed complementarity problems”, Optimization Methods and Software 5 (1995) 319. https://doi.org/10.1080/10556789508805619.
[4] K. Meintjes & A. P. Morgan, “A methodology for solving chemical equilibrium systems”, Appllied Mathematics and Computation 22 (1987) 333. https://doi.org/10.1016/0096-3003(87)90076-2.
[5] J. K. Liu & S. J. Li, “A projection method for convex constrained monotone nonlinear equations with applications”, Computers and Mathematics with Appllications 70 (2015) 2442. https://doi.org/10.1016/j.camwa.2015.09.014.
[6] Y. Xiao & H. Zhu, “A conjugate gradient method to solve convex constrained monotone equations with applications in compressive sensing”, Journal of Mathematical Analysis and Applications 405 (2013) 310. https://doi.org/10.1016/j.jmaa.2013.04.017.
[7] V. M. Solodov & A. N. Iusem, “Newton-type methods with generalized distances for constrained optimization”, Optimization 41 (1997) 257. https://doi.org/10.1080/02331939708844339.
[8] Y. B. Zhao & D. Li, “Monotonicity of fixed point and normal mappings associated with variational inequality and its application”, SIAM Journal of Optimization 11 (2001) 962. https://doi.org/10.1137/S1052623499357957.
[9] M. V. Solodov & B. F. Svaiter, “A globally convergent inexact Newton method for systems of monotone equations”, in Reformulation: Nonsmooth, Piecewise Smooth, Semismooth and Smoothing Methods, M. Fukushima, L. Qi (Eds.), Kluwer Academic Publishers,
Boston, MA: Springer US., 1998, pp. 355-369. https://doi.org/10.1007/978-1-4757-6388-1_18.
[10] C. G. Broyden, “A class of methods for solving nonlinear simultaneous equations”, Mathematics of Computation 19 (1965) 577. https://doi.org/10.1090/S0025-5718-1965-0198670-6.
[11] M. K. Dauda, M. Mamat, M. A. Mohamed & M. Y. Waziri, “Improved quasi-Newton method via SR1 update for solving symmetric systems of nonlinear equations”, Malaysian Journal Fundamental and Appllied Sciences 15 (2019) 117. https://doi.org/10.11113/mjfas.v15n2019.1085.
[12] J. M. Ortega & W. C. Rheinboldt, Iterative solution of nonlinear equations in several variables, New York: Academic Press, 1970. https://doi.org/10.1137/1.9780898719468.
[13] J. E. Dennis & R. B. Schnabel, Numerical methods for unconstrained Optimization and nonlinear equations, Englewood Cliffs, NJ: PrenticeHall, 1983. https://doi.org/10.1137/1.9781611971200.
[14] S. Babaie-Kafaki & R. Ghanbari, “A descent family of Dai-Liao conjugate gradient methods”, Optimization Methods and Software 29 (2013) 583. https://doi.org/10.1080/10556788.2013.833199.
[15] R. Fletcher & C. Reeves, “Function minimization by conjugate gradients”, The Computer Journal 7 (1964) 149. https://doi.org/10.1093/comjnl/7.2.149.
[16] R. Fletcher, Practical method of optimization Volume 1: Unconstrained optimization, 2nd ed., Wiley, New York, 1997. https://doi.org/10.1002/9781118723203.
[17] Y.H. Dai & Y. Yuan, “A nonlinear conjugate gradient method with a strong global convergence property”, SIAM Journal of Optimization 10 (1999) 177. https://doi.org/10.1137/S1052623497318992.
[18] M. R. Hestenes & E. L. Stiefel, “Methods of conjugate gradients for solving linear systems”, Journal of research of the National Bureau of Standards 49 (1952) 409. https://doi.org/10.6028/JRES.049.044.
[19] E. Polak & G. Ribi´ere, “Note Sur la convergence de directions conjugees”, ESAIM: Mathematical Modelling and Numerical Analysis 3 (1969) 35. https://doi.org/10.1051/m2an/196903R100351.
[20] B. T. Polyak, “The conjugate gradient method in extreme problems”, USSR Computational Mathematics and Mathematical Physics 9 (1969) 94. https://doi.org/10.1016/0041-5553(69)90035-4.
[21] Y. Liu & C. Storey, “Efficient generalized conjugate gradient algorithms Part 1: Theory”, Journal of Optimization Theory and Applications 69 (1991) 129. https://doi.org/10.1007/BF00940464.
[22] Y. Huang & C. Liu, “Dai-Kou type conjugate gradient methods with a line search only using gradient”, Journal of Inequalities and Applications 66 (2017) 2017. https://doi.org/10.1186/s13660-017-1341-z.
[23] X. Y. Wang, X. J. Li & X. P. Kou, “A self-adaptive three-term conjugate gradient method for monotone nonlinear equations with convex constraints”, Calcolo 53 (2016) 133. https://doi.org/10.1007/s10092-015-0140-5.
[24] P. P. Gao & C. J. He, “An efficient three-term conjugate gradient method for nonlinear monotone equations with convex constraints”, Calcolo 55 (2018) 53. https://doi.org/10.1007/s10092-018-0291-2.
[25] L. Zhang, W. Zhou & D. H. Li, “Global convergence of a modified Fletcher-Reeves conjugate gradient method with Armijo-type line search”, Numersche Mathematik 104 (2006) 561. https://doi.org/10.1007/s00211-006-0028-z.
[26] L. Zhang, W. Zhou & D. Hui-Li, “A descent modified Polak−Ribiere−Polyak conjugate gradient method and its global convergence”, IMA Journal Numerical of Analysis 26 (2006) 629.
https://doi:10.1093/imanum/drl016.
[27] L. Zhang, W. Zhou & D. Li, “Global convergence of a modified Fletcher-Reeves conjugate gradient method with Armijo-type line search”, Numerische Mathematik 104 (2006) 561. https://doi.org/10.1007/s00211-006-0028-z.
[28] S. Babaie-Kafaki, R. Ghanbari & N. Mahdavi-Amiri, “Two new conjugate gradient methods based on modified secant equations”, Journal of Computational and Appllied Mathematics 234 (2010) 1374. https://doi.org/10.1016/j.cam.2010.01.052.
[29] W. Cheng, “A PRP type method for systems of monotone equations”, Mathematical and Computer Modelling 50 (2009) 15. https://doi.org/10.1016/j.mcm.2009.04.007.
[30] J. K. Liu, J. L. Xu & L. Q. Zhang, “Partially symmetrical derivative free Liu-Storey projection method for convex constrained equations”, International Journal of Computer Mathematics 96 (2019) 1787. https://doi.org/10.1080/00207160.2018.1533122.
[31] B. S. He, H. Yang, S. L. Wang, ”Alternationg direction method with self-adaptive penalty parameters for monotone variational inequalites”,
Journal of Optimization Theory and Applications 106 (2000) 337. https:
//doi.org/10.1023/A:1004603514434.
[32] D. H. Li & X. L. Wang, “A modified Fletcher-Reeves-type derivative-free method for symmetric nonlinear equations”, Numerical Algebra, Control and Optimization 1 (2011) 71. https://doi.org/10.3934/naco.2011.1.71.
[33] J. Liu & S. Li, “Multivariate spectral projection method for convex constrained nonlinear monotone equations”, Journal of Industrial and Management Optimization 13 (2017) 283. https://doi.org/10.3934/jimo.2016017.
[34] Y.H. Dai & Y. Yuan, “A Nonlinear conjugate gradient method with a strong global convergence property”, SIAM Journal on Optimization 10 (1999) 177. https://doi.org/10.1137/S1052623497318992.
[35] D. R. Han & W. Y., “A new modified Goldstein-Levitin-Polyak projection method for variational inequality problems”, Computers and Mathematics with Applications 47 (2004) 1817. https://doi.org/10.1016/j.camwa.2003.12.002.
[36] K. Ahmed, M. Y. Waziri, A. S. Halilu, S. Murtala & J. Sabi’u, “Another Hager-Zhang-type method via singular value study for constrained monotone system equations with application”, Numerical Algorithm 96 (2024) 1583. https://doi.org/10.1007/s11075-023-01678-8.
[37] K. Ahmed, M. Y. Waziri, A. S. Halilu, S. Murtala & H. Abdullahi, “Signal and image reconstruction with a double parameter Hager-Zhang-type conjugate gradient method for system of nonlinear equations”, Numerical Linear Algebra with Applications 32 (2025) 1. https://doi.org/10.1002/nla.2583.
[38] K. Ahmed, M. Y. Waziri, A. S. Halilu & S. Murtala, “Two RMIL-type schemes with compressed sensing applications”. Optimization Methods and Software (2024) 1. https://doi.org/10.1080/10556788.2024.2425001.
[39] M. Y. Waziri, K. Ahmed & J. Sabi’u, “A Dai-Liao conjugate gradient method via modified secant equation for system of nonlinear equations”, Arabian Journal of Mathematics 9 (2019) 443. http://doi.org/10.1007/s40065-019-0264-6.
[40] M. Y. Waziri, K. Ahmed & J. Sabi’u, “Descent Perry conjugate gradient methods for systems of monotone nonlinear equations”, Numerical Algorithm 5 (2020) 763. https://doi.org/10.1007/s11075-019-00836-1.
[41] M. Y. Waziri, K. Ahmed, J. Sabiu & A. S. Halilu, “Enhanced DaiLiao conjugate gradient methods for systems of monotone nonlin ear equations”, SeMA Journal 78 (2020) 15. https://doi.org/10.1007/s40324-020-00228-9.
[42] J. Sabi’u, A. Shah & M. Y. Waziri, “Two optimal Hager-Zhang conjugate gradient methods for solving monotone nonlinear equations”, Appllied Numerical Mathematics 153 (2020) 217. https://doi.org/10.1016/j.apnum.2020.02.017.
[43] J. Sabi’u, A. Shah, M. Y. Waziri & K. Ahmed, “Modified Hager-Zhang conjugate gradient methods via singular value analysis for solving monotone nonlinear equations with convex constraint”, International Journal of Computational Methods 18 (2021) 2050043. http://doi.org/10.1142/S0219876220500437.
[44] M.Y. Waziri, H. Usman, A.S. Halilu, K. Ahmed, “Modified matrix-free methods for solving systems of nonlinear equations”, Optimization 70 (2020) 2321. https://doi.org/10.1080/02331934.2020.1778689.
[45] M. Y. Waziri & J. Sabi’u, “A Derivative-free conjugate gradient method and its global convergence for solving symmetric nonlinear equations”, Hindawi Publishing Corporation, Internation Journal of Mathematics and Mathematical Sciences (2015) 1 961487. https://doi.org/10.1155/2015/
961487.
[46] K. Ahmed, M. Y. Waziri, S. Murtala, A. S. Halilu & J. Sabi’u,
“On a scaled symmetric Dai–Liao-type scheme for constrained system of nonlinear equations with Applications, Journal of Optimization Theory and Applications 200 (2024) 669. https://doi.org/10.1007/s10957-023-02281-6.
[47] K. Ahmed, M. Y. Waziri, A. S. Halilu, S. Murtala, J. Sabi’u & H. Abdullahi, “Improved Dai-Yuan iterative schemes for convex constrained monotone nonlinear systems”, Mathematical Sciences 18 (2024) 707. https://doi.org/10.1007/s40096-024-00526-7.
[48] N. Ullah, A. Shah, J. Sabi’u, X. Jiao, A. M. Awwal, N. Pakkaranang, S. K. Shah & B. Panyanak, “A One-parameter memoryless DFP algorithm for solving system of monotone nonlinear equations with application in image processing”. Mathematics 11 (2023) 1221. https://doi.org/10.3390/math11051221.
[49] K. Sainia, M. Sainia, A. Kumara & D. K. Sainib, “Availability predictions of solar power plants using multipleregression and neural networks: an analytical study”, Journal of the Nigerian Society of Physical Sciences 7 (2025) 2398. https://doi:10.46481/jnsps.2025.2398.
[50] E. C. Ukekwea, A. A. Obayia, A. Johnson, D. A. Musab & J. C. Agboa, “Optimizing data and voice service delivery for mobile phones based on clients’ demand and location using affinity propagation machine learning” Journal of the Nigerian Society of Physical Sciences 7 (2025) 2109. https://doi:10.46481/jnsps.2025.2109.
[51] J. P. Olumuyiwa, S. F. Gerard, J. O. Agbaje & K. Oshinubi, “An empirical study on anomaly detection using density-based and representative-based clustering algorithms”, Journal of the Nigerian Society of Physical Sciences 5 (2023) 1364. https://doi.org/10.46481/jnsps.2023.1364.
[52] M. Y. Waziri, K. Ahmed & J. Sabi’u, “A family of Hager-Zhang conjugate gradient methods for system of monotone nonlinear equations”, Appllied Mathematics and Computation 361 (2019) 645. https://doi.org/10.1016/j.amc.2019.06.012.
[53] J. M. Perry, A class of conjugate gradient algorithms with a two-step variable-metric memory, Discussion Paper 269, Center for mathematical studies in economics and management sciences, northwestern university, evanston, IL, 1977.
[54] D. F. Shanno, “On the convergence of a new conjugate gradient algorithm”, SIAM Journal on Numerical Analysis 15 (1978) 1247. https://doi.org/10.1137/0715085.
[55] Y. H. Dai & C. X. Kou, “A nonlinear conjugate gradient algorithm with an optimal property and an improved wolfe line search”, SIAM Journal of Optimization 23 (2013) 296. https://doi.org/10.1137/100813026.
[56] S. S. Oren & E. Spedicato, “Optimal conditioning of self scaling variable metric algorithms”, Mathematical Programming 10 (1976) 70. https://doi.org/10.1007/BF01580654.
[57] S. S. Oren & D. G. Luenberger, “Self scaling variable metric (SSVM) algorithms, part I: criteria and sufficient conditions for scaling a class of algorithms”, Management Science 20 (1974) 845. https://doi.org/10.1007/BF00934764.
[58] M. Al-Baali, “Numerical experience with a class of self-scaling quasi Newton algorithms”, Journal of Optimization Theory and Application 96 (1998) 533. https://doi.org/10.1023/A:1022608410710.
[59] S. S. Oren, “Self scaling variable metric (SSVM) algorithms Part II: Implementation and experiments”, Management Science 20 (1974) 863. http://www.jstor.org/stable/2630095.
[60] Y. Huang & C. Liu, “Dai-Kou type conjugate gradient methods with a line search only using gradient”, Journal of Inequalities and Applications 66 (2017) 1.
https://doi.org/10.1186/s13660-017-1341-z.
[61] M. Al-Baali, “Numerical experience with a class of self-scaling quasi Newton algorithms”, Journal of Optimization Theory and Applications 96 (1998) 533. https://doi.org/10.1023/A:1022608410710.
[62] S. S. Oren, “Self scaling variable metric (SSVM) algorithms”, Part II: Implementation and experiments”, Management science 20 (1974) 863. https://www.jstor.org/stable/2630094.
[63] S. S. Oren & D. G. Luenberger, “Self scaling variable metric (SSVM) algorithms, Part I: Criteria and sufficient conditions for scaling a class of algorithms”, Management Science 20 (1974) 845. https://www.jstor.org/stable/2630094.
[64] S. S. Oren & E. Spedicato, “Optimal conditioning of self scaling variable metric algorithms”, Mathematical Programming 10 (1976) 70. https://doi.org/10.1007/BF01580654.
[65] M. Y. Waziri, K. Ahmed & A. S. Halilu, “A modified Dai-Kou-type method with applications to signal reconstruction and blurred image restoration”, Computational and Appllied Mathematics 41 (2022) 232. https://doi.org/10.1007/s40314-022-01917-z.
[66] K. Ahmed, M. Y. Waziri & A. S. Halilu, “On two symmetric Dai-Kou type schemes for constrained monotone equations with image recovery application”, Euro Journal on Computational Optimization 11 (2023) 1. https://doi.org/10.1016/j.ejco.2023.100057.
[67] Y. Narushima, H. Yabe, J. A. Ford, “A three-term conjugate gradient method with sufficient descent property for unconstrained optimization”, Journal of Optimization 21 (2011) 212. https://doi.org/10.1137/080743573.
[68] J. Zhang, Y. Xiao & Z. Wei, “Nonlinear conjugate gradient methods with sufficient descent condition for large-scale unconstrained optimization”, Mathematical Problems in Engineering 2009 (2009) 243290. http://dx.doi.org/10.1155/2009/243290.
[69] Y. H. Dai & L. Z. Liao, “New conjugacy conditions and related nonlinear conjugate gradient methods”, Appllied Mathematics and Optimization 43 (2001) 87. https://doi.org/10.1007/s002450010019.
[70] A. Y. Al-Bayati, W. H. Sharif, “A new three-term conjugate gradient method for unconstrained optimization”, Canadian Journal on Science and Engineering Mathematics 1 (2010) 108.
[71] W. Cheng, “A two-term PRP-based descent method”, Numerical Functional Analysis and Optimization 28 (2007) 1217. https://doi.org/10.1080/01630560701749524.
[72] N. Andrei, “A modified Polak-Ribi´ere-Polyak conjugate gradient algorithm for unconstrained optimization”, Optimization 60 (2011) 1457.
[73] J. Barzilai & J. M. Borwein, “Two-point step size gradient methods”, IMA Journal of Numerical Analysis 8 (1988) 141. https://doi.org/10.1093/imanum/8.1.141.
[74] M. Koorapetse, P. Kaelo & E. R. Offen, “A Scaled derivative-free projection method for solving nonlinear monotone equations”, Bulletin of the Iranian Mathematical Society 45 (2019) 755. https://doi.org/10.1007/s41980-018-0163-1.
[75] J. Yin, J. Jian, X. Jiang, M. Liu & L. Wang, “A hybrid three−term conjugate gradient projection method for constrained nonlinear monotone equations with applications”, Numerical Algorithm 88 (2021) 389. https://doi.org/10.1007/s11075-02-01043-z.
[76] Y. Ding, Y. Xiao & J. Li, “A class of conjugate gradient methods for convex constrained monotone equations”, Optimization 66 (2017) 2309. https://doi.org/10.1080/02331934.2017.1372438.
[77] W. L. Cruz, J. M. Martinez & M. Raydan, “Spectral residual method without gradient information for solving large-scale nonlinear systems of equations, Theory and experiments”, Mathematics of computation 75 (2006) 1429.
[78] W. L. Cruz, “A Spectral algorithm for large-scale systems of nonlinear monotone equations”, Numerical Algorithm 76 (2017) 1109. https://doi.org/10.1007/s1107s-017-0299-8.
[79] E. D. Dolan & J. J. More, “Benchmarking optimization software with performance profiles”, Mathematical Programming 91 (2002) 201. https://doi.org/10.1007/s101070100263.
[80] M. A. T. Figueiredo & R. Nowak, “An EM algorithm for wavelet-based image restoration”, IEEE Trans. Image Process 12 (2003) 906. https://doi.org/10.1109/TIP.2003.814255.
[81] E. T. Hale, W. Yin & Y. Zhang, “A fixed-point continuation method for ℓ1 regularized minimization with applications to compressed sensing”, SIAM Journal of Optimization 19 (2008) 1107. https://doi.org/10.1137/070698920.
[82] A. Beck & M. Teboulle, “A fast iterative shrinkage-thresholding algorithm for linear inverse problems”, SIAM Journal on Imaging Sciences 2 (2009) 183. https://doi.org/10.1137/080716542.
[83] M. Figueiredo, R. Nowak & S. J. Wright, “Gradient projection for sparse reconstruction, application to compressed sensing and other inverse problems”, IEEE Journal of selected topics in signal processing 1 (2007) 586. https://doi.org/10.1109/JSTSP.2007.910281.
[84] Y. Xiao, Q. Wang & Q. Hu, “Non-smooth equations based method for ℓ1 norm problems with applications to compressed sensing”, Nonlinear Analysis: Theory, Methods and Appl. 74 (2011) 3570. https://doi.org/10.1016/j.na.2011.02.040.
[85] J. S. Pang, “Inexact Newton methods for the nonlinear complementarity problem”, Mathematical Programming 36 (1986) 54. https://doi.org/10.1007/BF02591989.
[86] A. B. Abubakar, P. Kumam, H. Mohammed & K. Sitthithakerngkiet, “A modified Fletcher–Reeves conjugate gradient method for monotone nonlinear equations with some applications”, Mathematics 7 (2019) 1. https://doi.org/10.3390/math7080745.
[87] H. C. Raymond, C. Ho & M. Nikolova, “Salt-and-pepper noise removal by median type noise detectors and detail-preserving regularization”, IEEE Transactions on Image Processing 14 (2005) 1479. https://doi.org/10.1109/TIP.2005.852196.
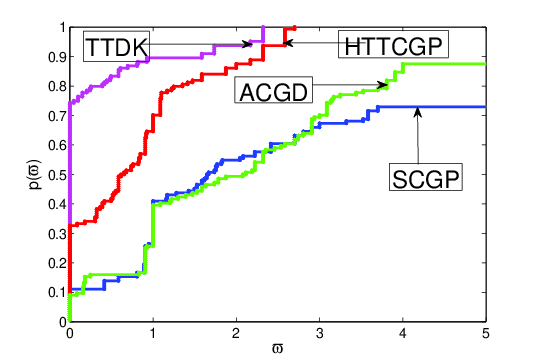
Published
How to Cite
Issue
Section
Copyright (c) 2025 K. Ahmed, M. Y. Waziri, A. S. Halilu, I. A. R. Moghrab, H. Abdullahi, S. M. Ibrahim, Y. B. Musa, S. Murtala, A. M. Awwal, A. Sambas

This work is licensed under a Creative Commons Attribution 4.0 International License.