Optimized aspect level sentiment analysis of tweet data using deep learning and rule-based techniques
Keywords:
Aspects Extraction , Sentiment Analysis, Deep Learning, Data SecurityAbstract
Social media platforms are no longer just for interacting with others and having fun; they are also now a place for people to voice their opinions on topics that are important to them. It has developed into a potent platform in recent years whereby elections can be won or lost. Governments and organizations are therefore becoming more and more interested in the opinions that citizens voice on social media platforms. Towards coming up with a localized sentiment analysis system, this research explored the use of rules and optimized Convolutional Neural Network (CNN) Deep Learning (DL) technique for aspect-level sentiment analysis of users’ tweets. A total of 26,401 tweets about the security situation in Nigeria were used as the test data. Most tweets in the Nigerian Twitter space are expressed in Pidgin language, which is a blend of English and local words, therefore, a rule-based technique was used to capture the local words and abbreviated character representations used in the Nigerian tweet data used for testing. Moreover, existing works have shown that CNN does not adequately capture modeling sequence information and long[1]distance dependency of texts. Therefore, this study employed existing word embedding to boost the performance of CNN for sentiment polarity classification. The proposed rule and optimized CNN model outperformed the traditional CNN and state-of-the-art Glove and word2Vec models with an accuracy of 89.31%, a recall value of 88.21%, a precision value of 88.15%, and an F1 score of 87.62%.
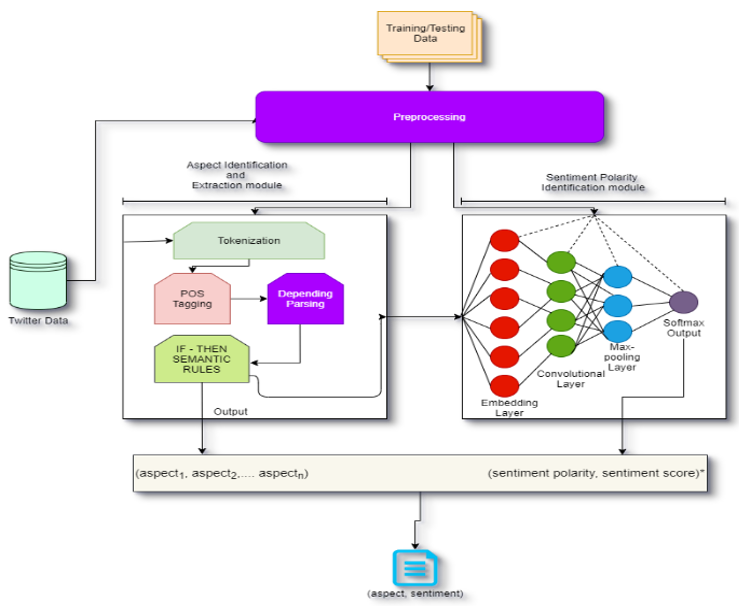
Published
How to Cite
Issue
Section
Copyright (c) 2025 S. N. Enemuo, O. N. Akande, M. O. Lawrence, I. C. Said

This work is licensed under a Creative Commons Attribution 4.0 International License.