Availability predictions of solar power plants using multiple regression and neural networks: an analytical study
Keywords:
Solar PV Power Plant, Artificial Neural Networks, Regression Model, Markovian ApproachAbstract
This analysis aims to develop an efficient mathematical model for prediction of the system availability of a solar photovoltaic power plant under the concept of redundancy and exponentially distributed random variables. For this objective, a stochastic model of the photovoltaic power plant is created with the help of the Markov birth-death technique. It is assumed that all the repairs are perfect and random variables statistically independent. The predictive techniques, namely regression analysis and artificial neural networks are used to predict the availability of the PV power plant in different experimental setups with the help of SPSS software. The impact of failure and repair rates on the availability of the PV power plant investigated. Experimental data used to calculate the Mean Absolute Error (MAE) and Root Mean Square Error (RMSE) of both predictive techniques. It is identified that the MAE and RMSE of the regression model are less in comparison to the ANN model. So, the regression model outperforms ANN in the performance prediction of PV power plants. The outcomes of this study may help design PV solar plants and plan maintenance strategies for solar PV power plants.
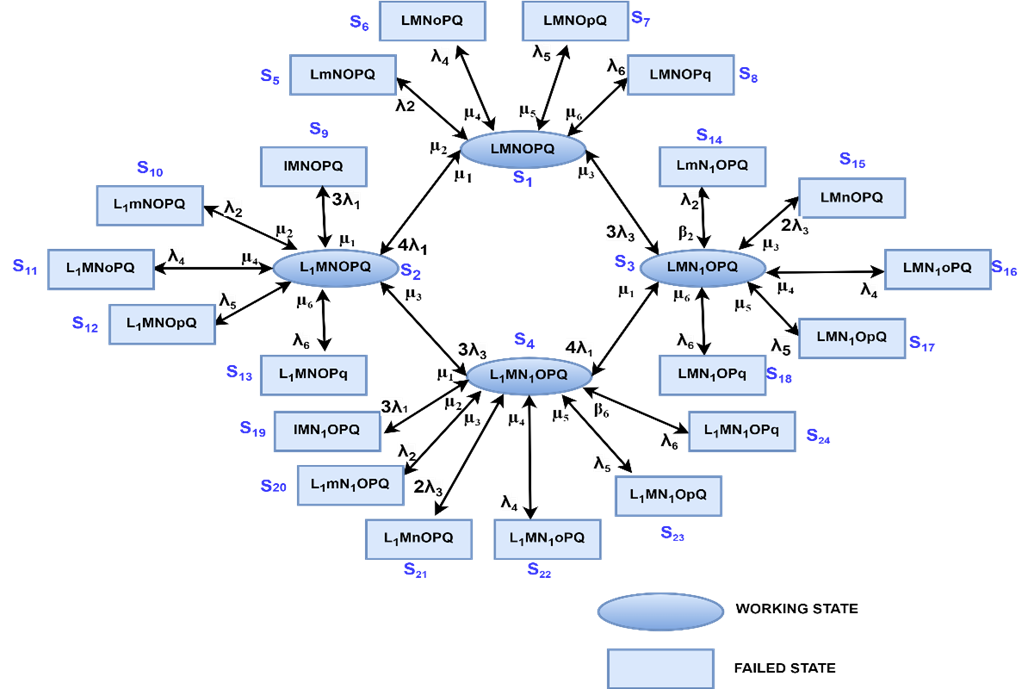
Published
How to Cite
Issue
Section
Copyright (c) 2025 Kanak Saini, Monika Saini, Ashish Kumar, Dinesh Kumar Saini

This work is licensed under a Creative Commons Attribution 4.0 International License.