Jackknife Kibria-Lukman M-Estimator: Simulation and Application
Keywords:
Jackknife Kibria-Lukman, M-estimator, Monte Carlo Simulation, Multicollinearity, Outliers, RobustAbstract
The ordinary least square (OLS) method is very efficient in estimating the regression parameters in a linear regression model under classical assumptions. If the model contains outliers, the performance of the OLS estimator becomes imprecise. Multicollinearity is another issue that can reduce the performance of the OLS estimator. This study proposed the Robust Jackknife Kibria-Lukman (RJKL) estimator based on the M-estimator to deal with multicollinearity and outliers. We examine the superiority of the estimator over existing estimators using theoretical proofs and Monte Carlo simulations. We put the estimator to the test once more using real-world data. We observed that the estimator performs better than the existing estimators.
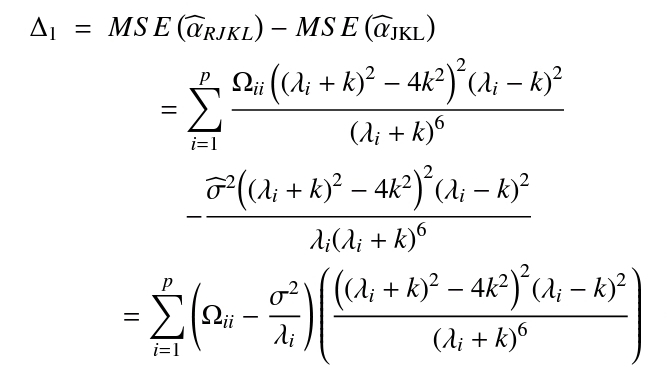
Published
How to Cite
Issue
Section
Copyright (c) 2022 Journal of the Nigerian Society of Physical Sciences

This work is licensed under a Creative Commons Attribution 4.0 International License.