Air quality prediction enhanced by a CNN-LSTM-Attention model optimized with an advanced dung beetle algorithm
Keywords:
Air quality prediction, DBO, CNN, LSTM, AttentionAbstract
Air pollution significantly impacts human health and socioeconomic development, making accurate air quality prediction crucial. This study proposes a hybrid CNN-LSTM-Attention model optimized with an improved Dung Beetle Optimization (IDBO) algorithm to enhance predictive performance. IDBO integrates multiple strategies to improve global search capabilities and overcome the limitations of conventional DBO. Experiments using PM2.5 data from Penang, Malaysia, demonstrate that the proposed model outperforms other models across multiple evaluation metrics R2 = 0.904, RMSE = 2.677, MSE = 7.168, MAE = 1.982, MAPE = 44.1% The findings validate the effectiveness of the proposed approach in improving air quality prediction, offering valuable insights for environmental monitoring and pollution control.
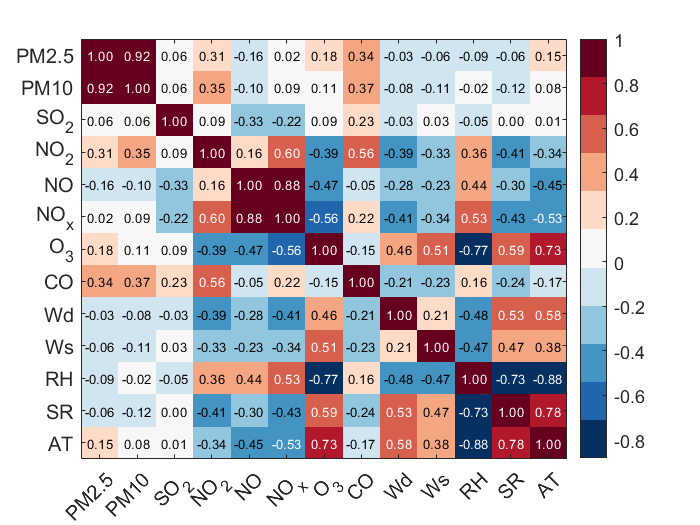
Published
How to Cite
Issue
Section
Copyright (c) 2025 xiaojie zhou, Majid Khan Majahar Ali, Farah Aini Abdullah, Lili Wu, Ying Tian, Tao Li, Kaihui Li

This work is licensed under a Creative Commons Attribution 4.0 International License.